Robert McKeon Aloe's Engineering Better Espresso: Data Driven Coffee has well exceeded its funding goal on Kickstarter with its unique data-dense angle on personal espresso improvement.
How to Make Better Espresso
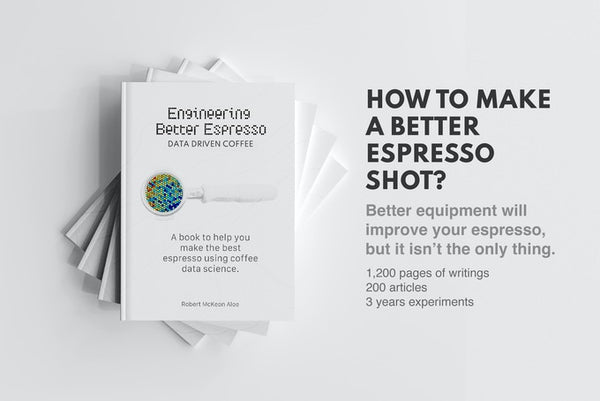
Espresso is the most unforgiving form of coffee with multiple variables that complicate the process. Information is available on the internet which allows someone new to espresso to learn how to get a decent shot. However, to go from good to the best is more ambiguous.
People think you have to upgrade coffee equipment for better espresso. Better equipment will improve your espresso, but it isn’t the only thing. There are plenty of cheaper ways and methods to improve espresso that can be done incrementally. Engineering Better Espresso: Data Driven Coffee is a book about improving espresso by incrementally improving each variable of the process using data science to help every espresso drinker regardless of their home or professional setup.
Robert's goal is to remove ambiguity and answer the question: How do I make a better shot?
Engineering Better Espresso
In this book, Robert focuses on four large areas of espresso, and for each one, he uses data to help give a deeper understanding of how to optimize parameters to produce better espresso.
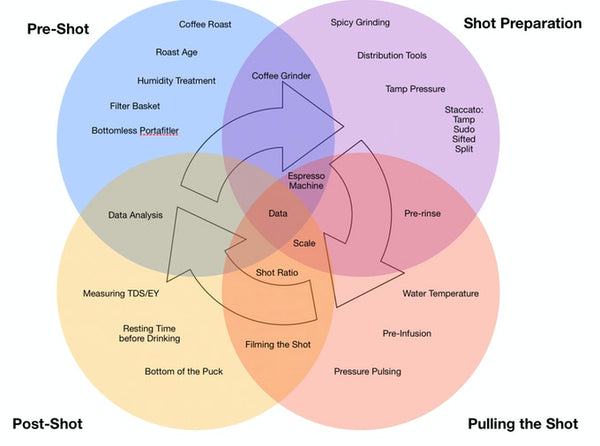
Some Data From Robert's Collection of Espresso Articles
This book pulls from nearly 200 articles written in the last three years in attempts to engineer better espresso. The research completed has allowed Robert to drastically improve his espresso shot and brings light to the possibility that there's still more to be discovered in espresso.
Filter Hole Analysis
This image shows the bottom of a spent espresso puck represented as Hue, Saturation, and Intensity. The bright spots in red would be darker brown in Red, Green, and Blue. These spots indicate where water flow was slower.
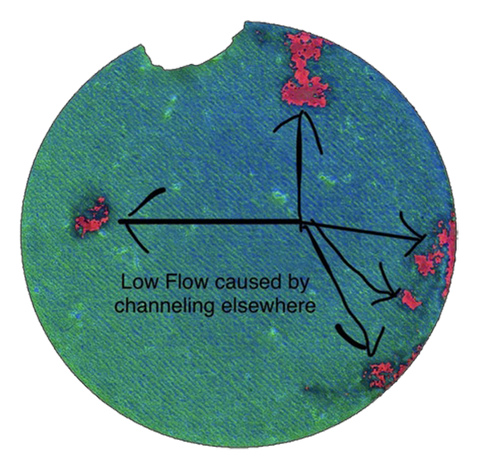
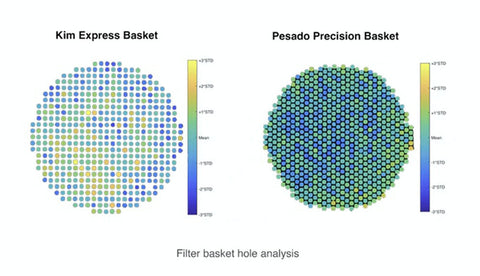
The Staccato Shot
Here is the design and development of the staccato shot. Fundamentally, this shot improves extraction and evenness of extraction because the layers are sifted. It's an advancement that levels the playing field of grinders and has brought Robert a better understanding of how coffee extracts.
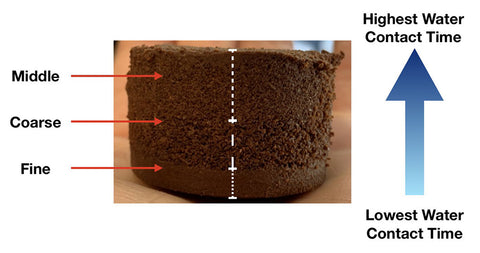
Tiger Stripes in Espresso
Robert analyzed tiger stripes in espresso using image processing to examine the variation by frame. Tiger stripes don’t correlate well to shot performance, and he sees them as a sign of channelling because they are streams of faster-moving, blonded coffee.
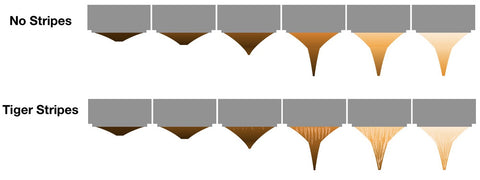
A Sample Page
The general layout of each chapter is to talk about a variable in terms of good, better, and best. This way you can see how to improve that variable for your setup. This philosophy is exemplified below with the focus topic being roast age.
About Robert
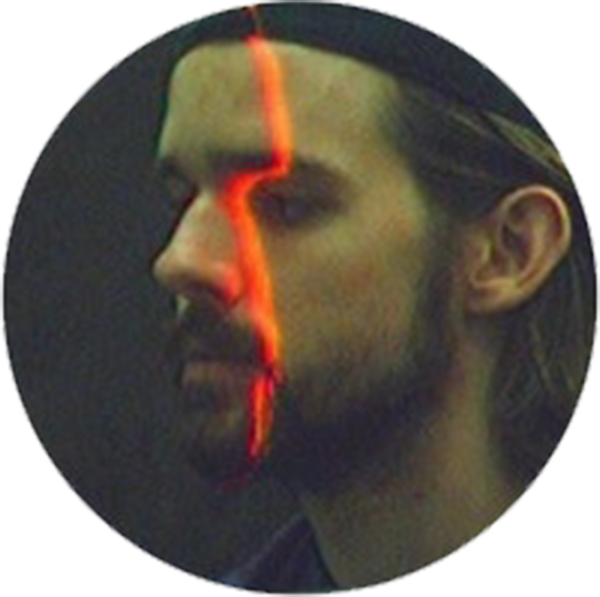
In 2006 he received a bachelor's and master's degree in Electrical Engineering at Detroit Mercy and extended his studies at the University of Notre Dame where he received his Ph.D. in Computer Science and Engineering.
His dissertation was focused on 3D facial recognition where he built a novel 3D face scanner by combining structured light with object tracking. He then worked extensively on recognition techniques using classical pattern recognition techniques.
He later worked four years at a startup doing 3D face recognition research before ending up at Apple. At Apple, Robert worked on wrist detection and background heart rate for the first Apple Watch, then Face ID, AR Kit, and People Detection for blind users.
Aside from coffee, most of his time is spent with his family, and he has a few hobbies like studying Italian, reading, gardening, building legos, running, snowboarding, and Disney.